Advanced driver-assistance systems (ADAS)
By Deepak Shankar, Mirabilis Design
Introduction
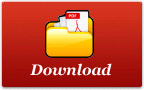
Autonomous Driver Assistance Systems (ADAS) are now an up surging technology in the automotive space. As these technologies become more pervasive, it becomes increasingly important for consumers to be informed about the systems on their vehicle and their functionality. However, several names marketed by producers, manufacturers, and the lack of consensus by industry regulatory groups, make it difficult for consumers to discern what features a vehicle has and how they actually work.
In recent years, the developments in traffic and technology caused an ameliorated interest in the analysis of interactions between road users. On highways, traffic intensity and traffic density increases rapidly. Therefore, drivers have to respond to each other to a higher extent and to adapt their driving etiquette rapidly to navigate other drivers. Several studies have dealt with this topic. Hence, there is a demand for understanding the behavior of driving and analyzing interactions between road users. Various research methodologies were developed to respond to this demand,
- Experimental runs with many real drivers in natural situations on real roads.
- Simulation of Driving by exposing a single human driver to simulated traffic.
- Traffic flow simulation that targets at predicting and simulating traffic situations under several conditions.
Each of these three methods has advantages and disadvantages. Anyhow, these conditions are complicated and uncontrollable for the test situations in real world traffic and the driving in safety critical situations is highly under risk because of ethical reasons.
With simulation in driving it is easy to predict the different traffic conditions on a human driver. This method is useful, because conditions can be controlled and desired test conditions can be generated. Furthermore, due to the simulated conditions, the realization of safety critical situations is possible. A disadvantage is that the interactions between drivers is limited, since the surrounding traffic is just simulated, not the real time input. Simulation of traffic flow makes it possible to scrutinize how single drivers influence other road users. So to generate the traffic flow simulation we use the VISUALSIM ARCHITECT to create the models and analyses a lot of vehicles in the same situation under controlled conditions.
THE STRUCTURE OF ADAS MODEL:
The major Five Components that power these Autonomous Driving Assistance System:
- Sensors
- Software
- Actuators
- Processors
- Mapping System
In general, a driver assistance system requires different sensors, an electronic control system in which Input devices such as sensors gather and respond to information and control a physical process by using electrical-energy in the form of an output reaction. And the loudspeakers receive the control signals and displays to warn the actuators to actively intercede in the vehicle control system. Current vehicle models are fitted with ultrasonic sensors which are used to measure the distance to the target by measuring the time between the emission and reception, basically used for parking assistance. Camera, Radar, and Lidar are the other sensors. Lidar similarly to radar sensors but emit light (light detection and ranging) Sensors continuously rotate and generate thousands of laser pulses per second. Automotive radars are used to perceive the speed and range of objects in the proximity of the car. An automotive radar consists of a receiver and a transmitter. The transmitter sends out radio waves that hit an object and bounce back to the receiver, determining the objects' distance, speed and direction. Radar and lidar are very well suited for measuring distances between your vehicle and objects in the environment. Also, these sensors can be used to determine relative speeds.
All sensor data is evaluated in ECU. In few vehicles, the steering wheel can also vibrate briefly, or a warning symbol appears on the speedometer display. In more complex systems some actuators intercede directly in the vehicle control system. In the meantime, Automatic Emergency Braking (AEB) assistance has become a legal requirement. The AEB system detects barricade, if the driver does not react in time, it automatically initiates emergency braking. Systems such as active lane departure warning or adaptive distance control, automatically control a vehicle's speed and the steering angle.
Software is considered the backbone of hardware functions. The wiring of the actuation system in vehicles has been a major conciliator of ADAS. This allows many ADAS systems to function normally with other electrical components. The processors analyze the data from the ADAS system and vehicle sensors that can make the resulting decision achievable by actuators. The actuator system supports all starting from electric power steering to autonomous acceleration and breaking. In ADAS applications, processors are used for everything from building a real time model of a car’s structure to proximity calculation and threat levels of the environment. The infrastructure and geographical information is collected, stored and updated via sensors to direct a vehicle’s exact location. This information is managed and sent to the control system even if the GPS coverage fails.
This Autonomous Driving Assistance System is built with 4 Radars, 6 cameras and 2 Lidars connected to 12 ECUs, gateways and IEEE802.1Q networks. The prototype is set up to quickly modify feature packages and hardware distribution to determine the hardware and network configuration. The response time for an active safety action is the primary criteria.
The below ADAS model is constructed using VISUALSIM Software
Figure 1: ADAS Model
The Graphs of the ADAS Model developed using VISUALSIM Software
Fig 2: Latency Plots
The latency observed at various stages of the model is shown in figure 2. The “Latency ADAS function” graph shows the observed latency for the incoming data from the Position Sensor and the Spark Knock Sensor. Position sensors are used in automobiles to determine the steering wheel position, pedal positions, seat positions, and the position of various valves, knobs and actuators. Whereas a knock sensor is essentially a small “listening” device in or on the engine that detects these irregular vibrations and sounds that come from the engine block. The knock sensor picks up vibration and sound coming from the engine block, turns it into an electronic signal and sends that signal to the engine control unit (ECU). The car’s computer then judges the information and determines whether or not ignition timing should be altered. The Latency ADAS function 2 graph shows the latency plots for the Coolant Temperature Sensor, Infrared Camera and the LIDAR whereas Latency ADAS function 3 graph depicts the latency observed in RADAR and the Vision Sensor. A coolant temperature sensor measures the temperature of the coolant mix in the cooling system, giving an indication of how much heat the engine is giving off. LIDAR, RADAR and Camera are the environment sensors central to the autonomous car operation. They used to detect and classify the objects around the car by location and velocity. Each of the sensors has limitations and the information obtained from them is fused together with confidence prior to making a decision on the vehicles trajectory.
Figure 3: Power Plot
Figure 4: VisualSim Architect- CAN Node Messages
Figure 3 depicts the power consumption plot where the blue line represents the instantaneous power consumption whereas the red represents the average power consumption. The CAN NODE Messages shows the debug messages generated from the CAN bus.
CONCLUSION :
ADAS systems have the embryonic to improve road safety across the globe. Today, ADAS is nearly conventional. Starting from the high-end models to the compact economy cars, they offer a wide array of facilities focusing on driver safety. With all the enhanced features and pleasure content that are available in a vehicle, the driver must still focus on driving. Sensor technology can help to keep a check on whether the driver is distracted or focused and sends the signal to the driver.
Mirabilis Design is Silicon-Valley, EDA Software Company providing solutions to accelerate innovation in electronics and semiconductors.
Bio of the authors
Deepak Shankar is the founder of Mirabilis Design, Mr.Shankar has over two decades of experience in management and marketing of system level design tools. Prior to establishing Mirabilis Design, he held the reins as Vice President, Business Development at MemCall, a fabless semiconductor company and SpinCircuit, a joint venture of industry leaders Hewlett Packard, Flextronics and Cadence. He started his career designing network simulators for the US Federal Agencies and managing discrete-event simulators for Cadence. His extensive experience in product design and marketing stems from his association with the EDA industry in multifaceted roles. An alumnus of University of California Berkeley with an MBA, he holds a MS in Electronics from Clemson University and a BS in Electronics and Communication from Coimbatore Institute of Technology, India.
If you wish to download a copy of this white paper, click here
|
Related Articles
- Advanced Packaging and Chiplets Can Be for Everyone
- Optimal OTP for Advanced Node and Emerging Applications
- Advanced Topics in FinFET Back-End Layout, Analog Techniques, and Design Tools
- Artificial Intelligence (AI) utilizing deep learning techniques to enhance ADAS
- The Role of Interconnection in the Evolution of Advanced Packaging Technology
New Articles
- Accelerating RISC-V development with Tessent UltraSight-V
- Automotive Ethernet Security Using MACsec
- What is JESD204C? A quick glance at the standard
- Optimizing Power Efficiency in SOC with PVT Sensor-Assisted DVFS Technology
- Bandgap Reference (BGR) Circuit Design and Transient Analysis in 90nm VLSI Technology
Most Popular
![]() |
E-mail This Article | ![]() |
![]() |
Printer-Friendly Page |