Understanding mmWave RADAR, its Principle & Applications
By Snehal Buche, eInfochips
Introduction:
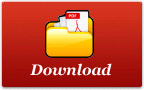
What Is RADAR? Radio Detection and Ranging is a system or device that uses electromagnetic radio waves to determine the range, velocity, and angle of the objects in the surrounding area. This system transmits an electromagnetic wave signal, which is reflected by an object in the path. By capturing this reflected signal and with some signal processing, RADAR systems can determine the range, velocity, and angle of the interested objects. These attributes of RADAR treat them as sensors, often called RADAR sensors.
A complete RADAR system comprises a transmitter (TX) and receiver (RX) of radio frequency (RF) components; analog components for clocking; and digital components such as Analog to Digital Converters (ADCs), microcontrollers (MCUs) and Digital Signal Processors DSPs. Even after having such usability, RADAR did not find application in any domain other than weather, remote sensing, and satellites. The major reason for this being the need to design a high-precision RADAR with low cost and low power consumption. The latest improvement in semiconductor technology has led the RADAR’s discrete components to be more cost and power effective with improved precision and configurability. The use of a short wavelength like the millimeter wave (mmWave) from the electromagnetic spectrum in the RADAR gives advantages of smaller antennae and other device form factors, which often integrate the whole RADAR system in a chip. This advancement has spurred applications, research, and developments in wireless sensing and finding engineering solutions for different domains using mmWave RADAR sensors.
Besides RADAR, there are many remote or wireless sensing options such as camera, Light Detection and Ranging (LiDAR), and ultrasonics, but these sensors have operational range limitation, environmental limitation, and some of them are expensive. RADAR can offer better solutions to them. Also, various privacy laws restricting the use of common sensors like cameras. However, RADAR lacks object classification and has sparse detection. The advent of Machine Learning (ML) and Artificial Intelligence (AI) with new estimation techniques and the concept of Multiple-Input Multiple-Output (MIMO) has improved RADAR application greatly to act as a standalone sensor or complement fusion sensor. restrict uses of common sensors like cameras. On the other hand, RADAR lacks object classification and has relatively sparse detection. The advent of Machine Learning (ML) and Artificial Intelligence (AI) with new estimation techniques and the concept of Multiple-Input Multiple-Output (MIMO) has improved RADAR application greatly to act as a standalone sensor or complement fusion sensors.
With the market development, the scope of application of the mmWave RADAR has expanded beyond the automotive field, gradually to smart cities, building automation, health monitoring, and other industries. Markets and Markets’ Recently released data shows by 2023, the total market in millimeter pod sensors will reach $20.6 billion. Car RADAR is the main driving force of this wave of growth, but with the Internet of Things (IoT), the healthcare market is expected to become another wheel that drives the millimeter wave RADAR market.
Applications:
- Automotive Applications
4D mmWave RADAR for multi-object tracking device in advanced driving assistant systems (ADAS), for driving safety, collision detection, and parking aids. - Medical Applications
mmWave RADAR can detect and monitor human gestures, emotions, movements, blood circulation, and heartbeat along with offering some privacy. - Robotics Applications
Used for imaging and sensing, object detection, navigation, and vision. - Meteorological Applications
mmWave RADAR helps in cloud analysis, crop analysis, radiometry, GIS, and climate study. - Aerospace and defense
Missile interception and guidance, UAV, airborne ranging, stealth operations, and flight controls. - Industrial and Automation Application
For quality control, crack detection, liquid and volumetric flow detection, leakages, and material classification. - Civilian applications
Used in air traffic control systems, low-altitude space surveillance, risk avoidance early warning system, surveillance in debris area or snowy area, smart city, IoT, and intelligent home.
In the following section, we will review and understand the technical aspects, application aspects, some mmWave RADAR concepts, estimation techniques, and MIMO concept. We will also look at different implementation platforms for RADAR estimation DSP part.
mmWave RADAR is a non-contact sensor, working in a spectrum of 10 millimeters (30 GHz) to 1 millimeter (300 GHz). This offers accurate location sensing, velocity, and angle, and can be performed with no interference. mmWave RADAR transmits pulse signals and detects targets from the reflections it receives through an array of antennas. Further processing estimates the distance to the target, angle of arrival, and the relative velocity. Currently, there are three major working bands - 24GHz millimeters, 77GHz millimeter, and 60GHz millimeter wave RADAR. 24GHz millimeter wave RADAR is cheap but has lesser bandwidth (250MHz) limiting application and accuracy. The 77GHz band has a high bandwidth of 4GHz, gives higher resolution/accuracy thus picking up for 24Ghz band. The 60GHz mm RADAR with 7GHz bandwidth used for short-range applications with high precision also offers a smaller system design. The 60GHz band is expected to become the mainstream of the intelligent homes market and health monitoring.
Advantages of millimeter wave RADAR:
- Small antenna caliber: Narrow beam gives high tracking, accuracy; high-level resolution, high-resistance interference performance of narrow beam; high antenna gain; smaller object detection.
- Large bandwidth: High information rate, details structural features of the target; reduces multipath, and enhances anti-interference ability; overcomes mutual interference; high-distance resolution.
- High Doppler frequency: Good detection and recognition ability of slow objectives and vibration targets; can work in snow conditions.
- Good anti-blanking performance: Works on the most used stealth material.
- Robustness to atmospheric conditions such as dust, smoke, and fog compared to other sensors.
- Operation under different lights: RADAR can operate under bright lights, dazzling lights, or no lights.
- Insusceptible to ground clutter: Allowing for close-range observations. The low reflectivity can be measured using mmWave RADAR.
- Fine spatial resolution for the same range, mmWave RADAR offers finer spatial resolution than microwave RADAR.
Limitations of millimeter wave RADAR:
- Line of sight operation.
- Affected by water content, gases in environment.
- Affected by contaminated environment and physical obstacles.
mmWave RADAR sensor components:
Figure 1. mmWave RADAR sensor basic block
The above diagram shows the basic structure of the mmWave RADAR sensor blocks which are mostly used across all applications. They are described as per below:
DAC or waveform generator:
In RADAR, signals are transmitted as pulse frequency modulated signals. RADAR uses Frequency Modulated Continuous Wave (FMCW), a continuous varying frequency signal modulated at a known rate over a fixed time period. Normal Continuous Wave (CW) signal lacks marker and time estimation of range and velocity calculation, whereas in FMCW even small-time difference creates a phase shift for estimation. Whereas pulsated signal helps in discrete signals on the same TX and RX array with more discernible echo. Length and repetition time and gap for this pulse defines the application and scope in mmWave RADAR. Also, it reduces power consumption of RADAR and helps in controlling interference and anti-jamming.
Due to the above characteristics, RADAR uses signal often termed as Chirps. Chirps' signals are frequency modulated short length signal where frequency follows either increasing or decreasing order of frequency of signal. It is transmitted discretely. In some applications, a bipolar golay code can be used for transmission for better correlation and resolution (reference 1,2)
Figure 2. Chirp signal and transmission as pulse
In RADAR, for the above desired signal, certain waveform generators can be used, but in this digital world more appropriate ways are to generate signal from Digital to Analog Converter (DAC), where signal of any sort can be stored in the digital form as Lookup for table. This can be then transmitted at a desired rate by using correct sample rate DAC. Gain, Signal to Noise Ratio (SNR), data rate for this transmission can be easily controlled by a central processing system of RADAR like MCU, FPGA (Field Programmable Gate Array), and DSP, for fine tuning and more control on the signal by configuring DACs. Nowadays, DACs are available at high data and sampling rates with multi-channel support, so that same device can be used for multiple transmissions.
Frequency Converters:
mmWave RADAR uses frequency in 30Ghz ~ 300Ghz range, which is not achievable at the highest DAC sampling rate and is very costly. For this purpose, normal DACs can be followed by an upconverter which will do FMCW modulation using carrier generated by a local oscillator, making signal in mmWave range with noise improvement offered by modulation. This provides a low-cost solution and more control and varied options on transmission as these oscillators, modulation, and upconverter can be configured by the central processing system of the RADAR, making the RADAR sensor configurable as per the application and scope.
Below are some modulation patterns used for different measurement purposes:
- Sawtooth modulation: Relatively large range (maximum distance) with a negligible influence of Doppler frequency.
- Triangular modulation: Better phase resolution.
- Square-wave modulation: Precise range estimation within objects but for lesser distances.
- Stepped modulation: For interferometric measurements.
- Sinusoidal modulation: Mostly easy to produce and has versatile uses.
Similarly, on the receiver side is a downconverter or demodulator using the same local frequency for extracting information signal from the carrier. As a result, we get over original reflected signal with changes as per echo for estimation. Being on smaller frequency, this estimate becomes easier on computation and processing. Again, these are configurable devices and all being on the same RADAR sensor gives us the option of optimal tuning of sensor and noise improvement. Many frequency converters are available with low-cost optimal solutions.
Beamformers:
In mmWave RADAR for good estimation we need accurate and known direction of transmission, field of view or field of target detection and can be any size in 3D space. It is necessary to limit the space in the desired and targeted application often called as point cloud frame. So, it is necessary for transmitting our chirp signal in a specific direction with specific power. Beamformer devices are used for this purpose. Beamformer nowadays provides multi-channel support so that we can transmit multi chirps with various transmission angles. This helps to scan a target point cloud frame. Beamformer can produce narrow beams with lesser side lobes, ensuring increased signal to noise power and improved signal to interference. Narrow beams in a band reduce interference to other bands and improve resolution of estimation from the RADAR. Alongside, the beamformer helps controlling the field of view of the RADAR and control of phase of transmission for multi object detection/resolution. Similar beamformer can be incorporated at RX side for more tuned echo capture as per TX, reducing noise. They ensure required gain and correction in echo signal. The beamformers available are also configurable from the central processing system of the RADAR for more controlled and wider application use of the RADAR sensor.
Figure 3. RADAR Point cloud frame example in 3D space
Antennae Arrays:
An antenna array is a single TX or RX connected to multiple antennaes working as a single antenna for radio waves. In mmWave RADAR, this array of antennas is connected in a phase shifted manner to beam or receive different phase shifter chirps or echo signals. This helps in controlling the RADAR resolution. Multi antennae also produce multi-TX systems for improving the RADAR functionality controlling power beam formation and multi object detection. This forms the basis of MIMO implementation of mmWave RADAR which we will review in the following sections. Required phase array requires appropriate layout and fabrication on PCB (Printed Circuit Board) for resonant matching and high reception in mmWave band. Being mmWave, this design requires smaller footprint antennae on PCB which is one of the advantages of mmWave RADAR.
Analog to Digital Converters (ADCs):
The final part on the RX side of the RADAR forms an ADC which helps to convert the analog form of chirps echo to digital part, which can be further processed by digital systems like MCU, DSP, and FPGA. Being in digital format, it helps in implementing noise reduction logic, signal processing, and computation. Resolution of ADC controls the accuracy and quantization of RADAR estimation calculation. ADC resolution can define the scope of your RADAR sensor application and they introduce a quantization noise. Currently, available ADCs has a higher resolution and data interface rate for high-speed processing of the RADAR in real-time.
Figure 4: Animation showing how a phased array works. (source Wikipedia)
.
RADAR processing system:
RADAR processing system involves processing and computation of range, velocity, azimuth, and angle of the identified objects. There are multiple techniques for estimation used in RADAR like zero crossing, Hilbert transform, and the most used Fast Fourier transform (FFT) for phase and angle estimation. Maximum likely hood estimation, range FFT, and autocorrelation are used for range estimation. MIMO based Doppler FFT, and correlators are used for velocity estimation. The starting point of the estimation starts with finding similarity or relation between the transmitted chirp and echo chirp, which is often done by different correlation algorithms or by frequency mixing/multiplication of TX and RX signal to get an Intermediatory Frequency (IF). It directly shows the relation between the TX and RX signals, which are further processed by a different algorithms like FFT.
The processing usually involves many linear and quadratic operations using exponential, floating, or larger size data. These can be computation intensive and require more processing for more accuracy. Along with that, different environmental or design limitation factors can introduce false estimation or noise, for which AI/ML and Artificial Neural Networks (ANN) comes into the picture. These algorithms are trained and optimized to further improve the RADAR estimation and prediction. This is what has improved the overall application and scope of the mmWave RADAR. But these algorithms are also multi-layered with complex computation.
The last part of this system can be a database, repository, base station or a platform like cloud, central server, rule, or a decision-based model, or multi-sensor fusion networks. These can help for cloud based remote processing application of RADAR, multi-node sensor fusions networks, remote or storage database, and IoT network, which alleviates the scope of RADAR from local application to global and multidomain. These are some fundamental needs of the current technologies. There are multiple platform options for having an intelligent and adaptive embedded RADAR processing system which exploits the progressive and pervasive ML and AI system architectural design. Below are a few options:
- Microcontrollers (MCUs): Microcontrollers are a system of processors with different peripheral and memory, which are used in combination to interface and configure different components of mmWave RADAR system. High frequency core helps faster computation of estimation algorithms and AI/ML logic. Also, they support any programming framework like C/C++, Scala, Java, Python, or any other new languages giving fast design space exploration and running your applications. Running smarter Operating System (OS) software on Microcontrollers gives the RADAR system a complete embedded device definition. Running computationally intensive RADAR processing on Microcontrollers which already have many non-RADAR processes in the pipeline is a bottleneck. They are limited by scope in increasing their operating frequency and the number of CPU cores. Also, when multi-RADAR sensor device, components or custom implementation are there, they lack. They are not the optimal platform for intensive AI/ML code. As a result, MCU can limit the application scope of the RADAR.
- GPU: Graphical Processing Units (GPUs) are simpler processing units compared to CPUs but have more cores, resulting in extensive parallel processing.give high throughput for computational and process intensive algorithms, as a result, they are optimally suited for AI/ML code. Also, they are great for high floating-point calculations which is often needed of RADAR and AI/ML. However, GPUs require languages like CUDA and OpenCL, providing less flexibility of development environment. Further, they lack peripherals and independent core, limiting smart OS running on it. Often, they are used in combination with Microcontrollers (e.g., NVIDIA) where the MCU acts as the RADAR sensor configuration, central unit, and OS platform and GPU as offloading engine for the RADAR and AI/ML estimation and processing logic.
- TPU: TensorFlow Processing Units (TPUs) have been designed from the bottom up to allow faster execution of application. They perform fast dense vectors and matrix. Thus, are suited for applications dominated by matrix computations. That means they have lower flexibility compared to CPUs and GPUs. It is better to use them when computational models are based on TensorFlow. So, they cannot handle Custom RADAR sensor design and limit RADAR application.
- DSP: Digital signal processors are MCUs with optimized architecture for signal processing and computation, handling complex and high operation. They offer flexibility in the development environment and computation throughput between the CPU and GPU. They are accurate and fast on computation with reconfiguration flexibility. They can interface and configure RADAR systems. But they are limited to RADAR application scope due to complex programming (as computation are software driven), higher power usage, high cost, lack of portability within DSPs, and require a skilled engineer.
- FPGAs (Field Programmable Gate Array) are configurable and reprogrammable chips used to implement glue logic and custom functions. They form a powerful and strong contender for custom RADAR-based application solution. FPGA can be designed for many cores for parallel and heterogeneous computation for high throughput. Algorithm design from AI/ML to DSP can be programmed on FPGA which is hardware driven and more reliable. They offer custom and standard interface for different RADAR components, offering immense help for custom RADAR sensor design and application. Additionally, they offer validation and verification platform for your RADAR sensors. FPGA has soft MCUs or even hard MCUs like in System on Chip (SoC FPGA) which provides platform for smart OS and can be programmed with any language just like normal MCUs. That is why FPGA is increasingly being explored for more support, development, and & research activity on mmWave RADAR.
FPGAs have a hard DSP multiplier but are limited in quantity in relative to DSPs, so floating point calculations are limited. An optimal logic design and pipelining, converting floating point to integer operation can give equivalent accuracy as DSPs. They may lack CPU frequency, core as in MCU but their ability to offload and increase peripherals/interfaces (both standard and custom), parallel pipelining of process intensive task, surpasses that limitation. They can always translate software bottleneck processes into hard logic. Alongside they can interface external MCUs, DSP, and GPU for more flexibility. FPGA requires specialized engineer resources and HDL language like Verilog /VHDL. They now support C-based programming like HLS for embedded engineers, but optimized designs require an FPGA engineer. Apart from the cons, their decreasing cost and varied usability have already increased research and development not just in RADAR but for all modern technology including IOT (Internet of Things), AI/ML, and aerospace.
Apart from a multiple component RADAR sensor, many single chips or single module RADAR sensor solutions offered are also available in the markets and explored for faster RADAR-based product development. Below are a few options:
- https://www.arrow.com/en/research-and-events/articles/RADAR-solutions
- https://www.electronicspecifier.com/products/sensors/arrow-jorjin-team-up-to-develop-mmwave-RADAR-sensor
- https://www.ti.com/lit/pdf/swra554
- https://www.arrow.de/-/media/arrow/files/pdf/h/high-performance-integrated-24-ghz-fmcw-RADAR-transceiver-chipset.pdf?
mmWave RADAR estimations:
The basic estimation of mmWave RADAR starts from sending a signal pulse from the TX and then receiving its echo on the RX side. Computing or analyzing the relation between the TX and RX signal helps to estimate range, velocity, and azimuth/angle of target object. To start with, we send a pulse of FMCW signal called as chirp signal, for generality, we will refer to it as a sinusoidal frequency modulated chirp signal shown in Figure 5.a. This offers good noise reduction and a better quality of reception. For better noise immunity, a complementary golay signal can be implemented, which is for now out of scope for this paper.
So, consider chirp from Figure 5. a. if we make frequency modulation linear with time and plot frequency vs time, we get a plot as in Figure 5.b., Xplot shows the time period t of pulse and Yplot shows bandwidth B of our chirp (max frequency – min frequency). Slope S shows rate of change of frequency or even change of power spectrum over time. When chirp traverses through space and gets echoed back to RX, it acquires a phase shift and amplitude change keeping chirp frequency linearity the same. Thus, we can see RX chirps in Figure 5.c., where the difference in time t between the TX and RX chirps is time difference or time taken for chirp to echo back. Different distance objects take different time. We can define a time relation with phase φ of signal as below.
φ = 2πfc --- eqn 1
Now, let the object be at distance d, so chirp will traverse 2d distance in space, and velocity of EM waves is considered equal to the light speed c. We can say time taken tc will be as below.
tc = 2d / c --- eqn 2
Taking eqn 1 and 2, we can derive the relation of the phase as below, whereas λ is the wavelength of the chirp.
φ = 4πd / λ --- eqn 3
So, eqn 3 defines phase difference as linear with distance d, that means more the distance, more will be the phase shift. Thus, the length of the chirp or time period t and bandwidth B will define the max distance covered by the RADAR for range estimation, more chirp length means more distance can be covered reliably. Now, these chirps are transmitted periodically in gap of tg (Figure 5.d.), each chirp can be used for particular region of 3D space, so for a point cloud frame, N (number of chirp) will be required. Chirp gap tg is dependent on our frame rate, data rate handled by the RADAR processing system and should be minimum to accommodate all echo chirps of previous TX chirp. Multi TX antennae can produce multi chirps for different purposes and multi object detection.
Range Estimation:
As per object distance, we get a phase shifted chirp version. If frequency multiplication is done on the TX and RX signal, we get an IF tone signal, which is directly related to the phase of received signal or time overlapping of both signals. From Figure 6.a., we could see the IF tone created. Multiple target objects kept at different distances produce a different phase shifted echo signal, and eventually produce different IF tone as shown in Figure 6.b. This characteristic can be used for range estimation as per the equations below.
d = c/2Stc --- eqn 4
Where S is the slope of the frequency plot. Bandwidth B is given as B = Stc, so, the above eqn considers the small incremental distance Δd
Δd = c / 2B --- eqn 5
From the above equation it is clear that the small incremental d or the smallest resolution of range is function of inverse B, increasing bandwidth will increase the range resolution. Considering Ghz operation of mmWave RADAR, range resolution generally is in order of centimeters. Phased antennae array gives multi IF tone which can further be used in AI/ML based code for improving the range resolution of the RADAR.
Velocity Estimation:
For velocity estimation, a series of multi-chirp transmissions is often used. Suppose for a stationary object, back-to-back chirp produces nearly the same IF tone. Let us replace d from eqn2 in eqn3. Also consider velocity v in place of c, after rearranging, the resultant equation we get is:
v = λ φ /4πtc --- eqn 6
A small change in velocity Δv is related to change in phase Δφ, for multiple chirps, if the object is in motion, it gets the same frequency IF tone but is phase shifted and that phase shift is directly related to the velocity of the object. Again, this characteristic can be used to estimate the velocity of an object. The method will fail if multiple moving objects with different velocities are at the same distance from the RADAR. As they are at the same distance, we will have identical IF frequencies. This RADAR system must transmit more than two chirps, say a set of N equally spaced chirps. This is where phased antennae array comes into the picture. Each antenna sends consecutive chirps with small phase shifts due to their arrangement. This eventually gives the phase a variance in echo signal at the RX, which helps for multi object velocity estimation.
As per eqn6, we can say velocity estimation is inversely related to time, in this case of multiple chirps, it is related to time between the chirp as tg. Due to the periodic nature of signal v ~ λ /4tc, Vmax estimation can be done by reducing the time between the chirps, which can be termed as velocity resolution. If the time is considered for multiple chirps say a frame of chirp, then velocity resolution is dependent on the frame length tf. Phased antennae RX array with AI/ML based code can further improve the velocity resolution of the RADAR.
Angle Estimation:
Figure 7
For angle estimation, multiple antennae or antennae array are the most important. From Figure 7., we can see a system of one TX and two RX antennae. The chirp transmitted will echo back to two RX, but their distance traversed is different by Δd. Suppose the distance between the two antennae is l, by trigonometric function, we can say Δd = lsinϴ, here ϴ is the angle of arrival. Change in the distance gives a phase change in the echo signal at each receiver. These characteristics can be studied and used for angle estimations.
Let us replace Δd = lsinϴ in eqn3 and rearrange for getting equation for ϴ we get
ϴ = sin -1(λ φ / 2 πl) --- eqn 7
This approximates that change in Δϴ is nonlinear with the phase change Δφ but considering it for small angles sinϴ ~ ϴ the above relation becomes linear and hence can be exploited for angle estimation. Now for ϴmax, the maximum angular field of view can only happen if λ φ / 2 πl =1, the max angular field theoretically is 180 degree or π, taking φ = π we get l = λ / 2. This defines the optimal spacing between the antennae.
For angle resolution or for minimum angle separation estimation between two objects, we can rewrite the eqn 7 as ϴres = λ /Nlcosϴ and considering l = λ / 2 we get ϴres = 2/N. Here, N is the number of RX antennae. As stated, the angle resolution is inversely related to the antennae count. The more the antennae, the better the angle estimation. But increasing the number of the RX antenna can be limiting in some of the RADAR sensor designs. For this, the MIMO concept is utilized to enhance the RADAR application further.
MIMO RADAR:
MIMO RADAR system is a novel RADAR method in which MIMO stands for Multiple Input Multiple Output. It is a system of multiple antennae both on the RX and TX side. Each transmitting antenna radiates an arbitrary waveform independently of the other transmitting antennae. Each receiving antenna can receive these signals. Consider we want to improve the angle resolution, for that we implemented 4 RX antennae as per Figure 8. a. Here, one TX antenna transmits, and four RX antennae receive the chirps signals.
Similar implementations can be derived by an additional introduction of another TX antenna. In this setup, the first TX 1 transmits, and two RX antennae receive as per Figure 8.b. The next TX 2 transmits, and the two RX antennae receive as per Figure 8.c. Such Transmission effectively treat as 4RX antennae; hence such setup is called as 4 virtual antennae (2TX x 2RX) system. Using this, we increase the angle estimation and angle resolution with a lesser physical antenna.
Figure 8.a
Figure 8.b
Figure 8.c
Often in MIMO, different approaches are employed for chirp transmission in TX. One of the methods is TDM (Time Division Multiplexing). in this, consecutive multiple chirps are transmitted but those chirps are time shared, allotted to each TX one by one (Refer Figure 9.a). This method is easier to implement but the full capability of the sensor is not used, and power consumption is less. Other methods involve BPSK or binary phase shifting. Here, two TX as per Figure 10.b. are simultaneously transmitted but they are kept 180-degree relative phase shifted. Consider like +1chirp -1chirp or spatial separated chirps. This offers more utilization of sensors but for certain applications it can increase power consumption.
Figure 10.a
Figure 10.b
The MIMO concept with modern ANN and AI algorithms has evolved RADAR usage beyond the traditional application, which is trending research and development. This has, no doubt, introduced a larger application of mmWave RADAR.
Conclusion:
In this above review, we have explored the concept of mmWave RADAR sensor, its basic components, application scope, and the field of research and development. mmWave RADAR sensors have significant advantages compared to other sensors, making them an ideal engineering solution for varied domains. This article discussed the key processes and concepts of RADAR estimation, scope of integration of AI/ML, and IoT with mmWave RADAR sensor for modern technology uses and enhancement - both in standalone and fusion. The future of the mmWave RADAR technology is promising, with plenty of room for growth and expansion. Future advancements may result in smaller, more adaptable, and cost-effective industrial mmWave RADAR systems.
Einfochips’ hardware and software learning offerings help organizations build highly customized solutions across different technologies and domains. We have already entered research and development offers on mmWave RADAR with applications like automotive, ADAS system, healthcare, and IoT for different firms and companies. Einfochips has a strong competent team for mmWave RADAR H/W design development like PCBs, boards, and product design. Software team for embedded firmware and design development, application team and competent FPGA team for your custom engineer solutions on mmWave RADAR giving a platform for all engineering solutions. Together with ARROW, we cater and provide semiconductor sourcing and support for all your custom design and development needs. We have helped companies integrate FPGA and MCU/GPU architecture with HDL (Hardware Descriptive Language), Software (Linux driver and BareMetal) and H/W design (PCB and mechanical encasing) with sourcing. We deliver the utmost customer satisfaction and always gain a competitive edge over others.
Lastly, FPGA provides a stronger contender as a platform for a mmWave RADAR based custom solution / product. We will explore different implementation methods, techniques, logic for standard and custom signal processing, data acquisition and processing, control and configuration mechanism, and firmware support solutions for mmWave RADAR application in different technical papers to come.
Author Name: Snehal Buche
Author Bio: Snehal Buche is a Senior Engineer at Einfochips primarily focusing on FPGA embedded development. He currently has 3+ years of experience at Einfochips and overall 5.5+ years of experience on FPGA embedded development. Snehal has Post graduated as an Electronics Engineer. In free time, he likes to draw painting, sketches and do gardening.”
If you wish to download a copy of this white paper, click here
|
Related Articles
- Understanding Timing Correlation Between Sign-off Tool and Circuit Simulation
- Understanding the Importance of Prerequisites in the VLSI Physical Design Stage
- Understanding Interface Analog-to-Digital Converters (ADCs) with DataStorm DAQ FPGA
- Understanding Logic Equivalence Check (LEC) Flow and Its Challenges and Proposed Solution
- Dimensioning in 3D space: Object Volumetric Measurement by Leveraging Depth Camera-based Reconstruction on NVIDIA Edge devices
New Articles
- Why RISC-V is a viable option for safety-critical applications
- Dimensioning in 3D space: Object Volumetric Measurement by Leveraging Depth Camera-based Reconstruction on NVIDIA Edge devices
- What is JESD204B? Quick summary of the standard
- Post-Quantum Cryptography - Securing Semiconductors in a Post-Quantum World
- Analysis and Summary on Clock Generator Circuits and PLL Design
Most Popular
![]() |
E-mail This Article | ![]() |
![]() |
Printer-Friendly Page |